This is the third article in a three part mini-series on the topic of systematic trend investing. In part one we looked closer at what systematic trend investing is and why we do it. In the second part we discussed whether the sun has set for trend strategies or if they’re still viable.
There are multiple approaches to trend investing, the basic building blocks consists of three parts; initiation, holding, and liquidation.
When designing a trend strategy we must take into consideration and decide on parameters for:
- Instrument universe for providing diversification.
- Metric to use as trend indicator/signal/detection.
- Entry/exit rules for finding position state.
- Rebalancing frequency
- Time-frame horizon for holding the positions
- Risk management with regards to weighting scheme, stop-loss and/or take-gain.
Designing the systematic trend strategy
We divide the investment process into four sequential steps:
1. Create a “trend detection” methodology, also referred to as a signal
First we have to define how to detect that a trend has been established. This can be done using for instance a rolling confidence band (Figure 4). If the price moves out of the band, this will signal a trend.
2. Define a set of entry and exit rules to identify the current state
Secondly we have to decide when to act on the signal. A fully invested strategy will have either a long- or short position in all markets and takes a new long- or short position if the price crosses the upper- or lower band respectively.
3. Construct a balanced portfolio with regards to position sizing and risk management
The third step defines the size of each investment. The positions could for instance be equally weighted, or have equal marginal contribution to risk (by scaling with the inverse of the instruments volatility).
4. Follow-up by periodic rebalancing positions and rolling the futures contracts
The final step is the day-to-day operations comprising of futures rolling, portfolio rebalancing, trading flow, instrument due-diligence, and so on.
Diversifying the strategy for return-risk improvements
The return-risk improvements are often modest on individual instruments. A diversified trend strategy will typically use 40+ positions across a wide range of asset classes, markets, geographical regions, and sectors to diversify.
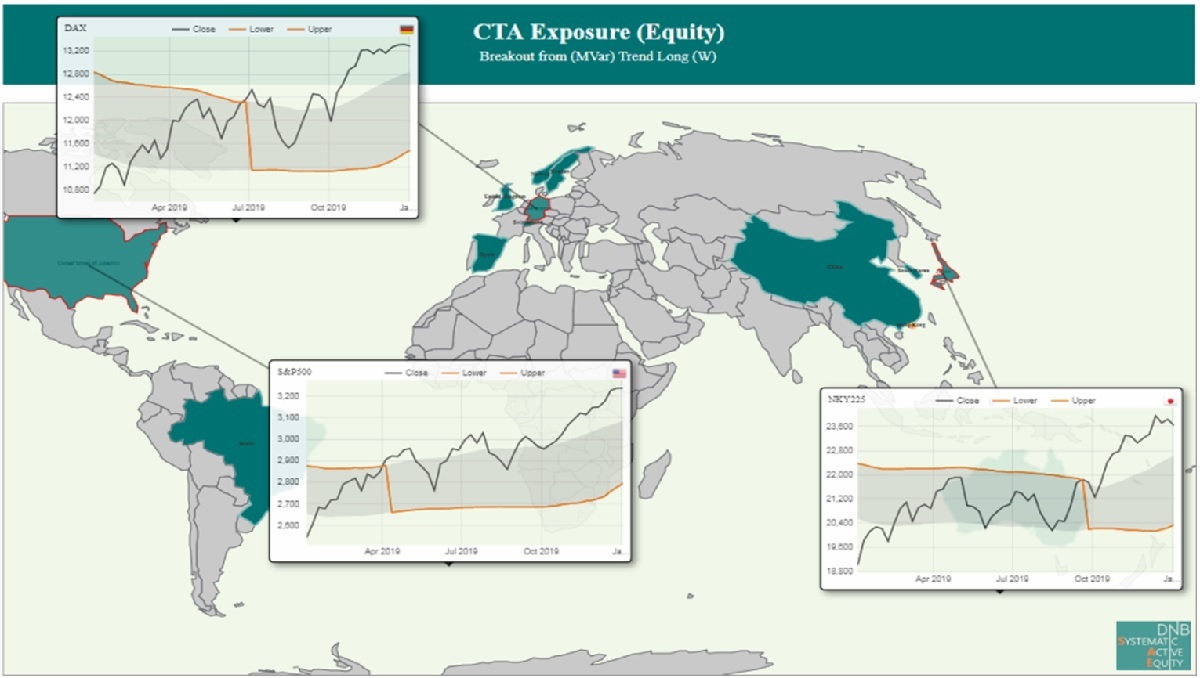
A momentum strategy can offer diversification across a wide variety of dimensions. We can include multiple signals like breakout from confidence band, and breakout from historical high/low.
Band = Mean ± SD
The signals can in addition have different time-frames, like a short-term high/low signal, combined with a long term confidence band signal. We can invest across multiple asset classes like currency, bond, equity, and commodity, include multiple markets within each asset class, and seek exposure within different geographies and sectors.
Implementing a time-series momentum trend-following strategy: An example
Let’s try to illustrate the theory with an example: Here we will use N instruments in the portfolio. As trend detection signal we will use a channel breakout strategy. For channel we will use the 52 weeks rolling 1 standard deviation band with weekly data granularity. This type of trend signal is known as a breakout from confidence band strategy. Mathematically, the rolling confidence band at time t for instrument n will be given by the formula[1]:

The strategy is to be fully-invested, thus all the positions will have either a long- or a short state. The entry/exit rule will therefore be defined as follows: Enter a long (short) position if the price[2] is above (below) the band. This strategy is illustrated in Figure 4 below. We then use this trend detection algorithm to find the current state for all N instruments in the portfolio.
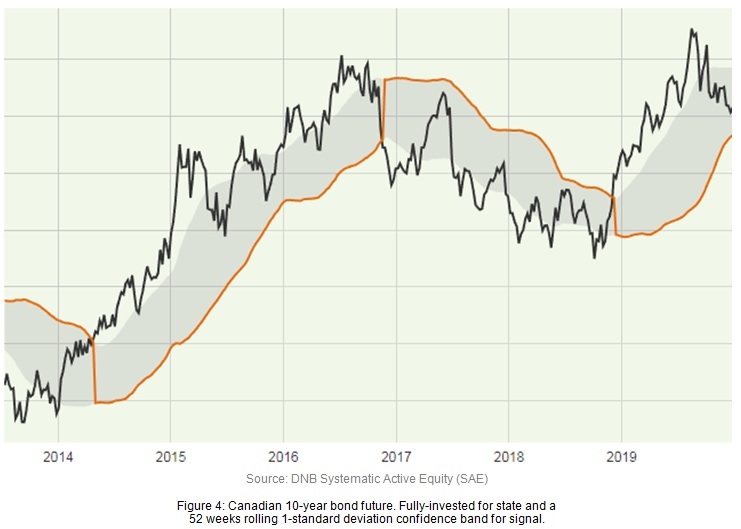
We now know the current state (long or short) for all our positions, but we need to construct a balanced portfolio, that is, to find the portfolio weights for all our positions. One approach is to let the instruments have equal marginal contribution to volatility, e.g. 15% annualized. We first calculate the 52 weeks annualized volatility[3] per instruments. We then find the portfolio weight at time t for each instrument n using the formula:

Portfolio rebalancing is performed weekly.
How do we take the strategy from idea into daily operation?
Though the basic idea behind systematic trend investing is fairly simple and transparent, implementing the strategy will require a large extent of proprietary configurations, as well as having a robust and efficient platform for monitoring and production.
At DNB, our diversified trend CTA strategies are maintained by the Systematic Active Equity (SAE) team within the Investments section in DNB Asset Management. Signal state calculations and portfolio constructions are implemented in MATLAB[4], which offers a safe, robust and efficient production platform.
Simplistic in design; large extent of proprietary configurations
All data, both configurations and calculation results are stored on a Microsoft SQL Server to ensure data integrity and quality. Tools for analyzing and monitoring signal state, contribution to risk/return, holding period, drawdown, futures rolling/rebalancing, and liquidity has been developed in HTML and JavaScript.
Key takeaway
Before launching a systematic CTA strategy it is important to analyzing the return-risk characteristics for the strategy in question. The back-test should be based on the actual implementation of the production environment. Historical data must be point-in-time and without any look-ahead biases. You should also include a live test-period where a fictive portfolio is rebalanced and traded accordingly. This to verify that all back-to-front systems are operational and that the day-to-day operations (like static futures data, futures rolling, and portfolio rebalancing) are handled correctly. A live test-period will also help to uncover any discrepancies in the back-test calculations.
Our long-term diversified trend CTA strategy delivered strong performance during 2019, especially from early February, and the strong performance has continued this year.
This period also illustrated many of the qualities that a diversified trend strategy has to offer, and also some of the risks. For currencies, the trend detection picked up a momentum in mid-2018, with a stronger US dollar against many of the major currencies, which overall contributed positively during the period. We experienced a very strong momentum in bonds from early March to mid-August, were a sharp turning-point lost some of the return. Equities had a short state entering 2019, and suffered from the strong equity markets during Q1, but for the total portfolio this was offset by the strong momentum in bonds, thus illustrating the importance of diversification. The positive momentum in equities was picked up, and contributed to performance when stocks rallied in Q4 and during the first half of January 2020. When equities experienced a sharp turning-point mid-January, due to the Coronavirus, the negative performance was offset by a stronger US dollar and falling yields.
This is the third and last article in a three part mini-series on the topic of systematic trend investing. The first part was: What is systematic trend investing?
[1] Means and standard deviations are calculated on the geometric cumulative return series (price) using equally weighted weekly data
[2] Based on the geometric cumulative return series
[3] Based on weekly log-returns using equally weighted data
[4] From MathWorks (https://se.mathworks.com/products/matlab.html)
Disclaimer: Nothing contained on this website constitutes investment advice, or other advice, nor is anything on this website a recommendation to invest in our Funds, any security, or any other instrument. The funds mentioned may not be available in the markets you represent. The information on this blog is posted solely on the basis of sharing insight to make our readers capable of making their own investment decisions. Should you have any queries about the investment funds or markets referred to on this website, you should contact your financial adviser.
Further reading
Below is a non-exhaustive list of relevant literature for further reading.
The cross-sectional momentum effect and The time-series momentum effect:
- Jegadeesh and Titman "Returns to Buying Winners and Selling Losers: Implications for Stock Market Efficiency" (1993)
- Erb and Harvey "The Tactical and Strategic Value of Commodity Futures" (2006)
- Miffre and Rallis "Momentum Strategies in Commodity Futures Markets" (2007)
- Asness, Moskowitz and Pedersen "Value and Momentum Everywhere" (2013)
- Menkhoff, Sarno, Schmeling and Schrimpf "Currency Momentum Strategies" (2012)
- The time-series momentum effect
- Burnside, Eichenbaum and Rebelo "Carry Trade and Momentum in Currency Markets" (2011)
- Moskowitz, Ooi and Pedersen "Time Series Momentum" (2012)
- Baltas and Kosowski "Momentum Strategies in Futures Markets and Trend-Following Funds" (2013)
- Ghia, Staal and Fattouche "Diversified trend following" (2014)
- Hurst, Ooi and Pedersen "A Century of Evidence on Trend-Following Investing" (2017)
Last updated:
Share: